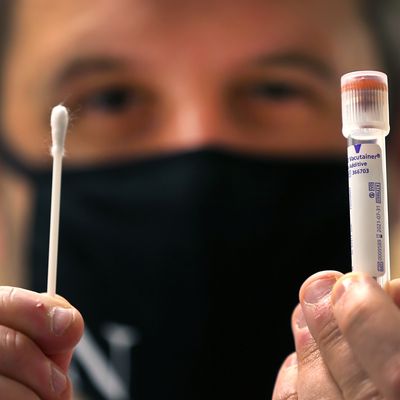
On November 18, Mayor Bill de Blasio announced he was temporarily closing the nation’s largest public-school system on the basis of one coronavirus statistic: the positivity rate. The city’s average rate exceeded 3 percent for the first time since June, which was taken to indicate that the virus’s spread could soon spiral dangerously out of control. Now, on December 7, de Blasio will reopen public elementary schools regardless of the fact that the city’s average positivity has climbed above 5 percent. The shift hints at the troublesome nature of a coronavirus statistic that heavily influences major decisions surrounding the pandemic in numerous states, counties, and school districts nationwide.
The number is deceptively basic. It’s the percentage of positive virus tests among all virus tests performed, both positives and negatives. It may reflect the level of disease transmission in a community, but a sudden rise in a particular location could mean an increase in infections coupled with a need for more testing of the general population, among whom the rate is probably lower. The positivity rate statistic grew popular this spring during an early, catastrophic lack of testing. As tests became more available, a large portion came back positive, indicating there was not enough testing to keep up with the explosive spread of the virus. In May, the World Health Organization recommended that governments use a positivity rate of 5 percent or lower lasting for two weeks as a threshold for reopening.
“From then on, there have always been these statements about the percent positivity,” says William Hanage, an associate professor of epidemiology at the Harvard Chan School of Public Health. “And really, it’s just a sort of post-traumatic stress disorder, focusing back on the early stages of the pandemic.”
The positivity rate began to be more widely used by officials and policy-makers to directly gauge the level of the coronavirus’s spread in a community, and that’s where things got sticky.
Under the current nonrandom, voluntary testing that prevails in the U.S., interpreting a positivity rate as an indicator of the spread of an infection is a little like assuming that a pond is well-stocked with fish after catching a few in a large mesh net swept through the water here and there. The positivity rate is an accurate indicator of spread in a community only if tests are taken by a group of people that is representative of an entire community, experts say.
But in nearly all U.S. cities and towns, tests are predominantly taken by people who feel sick, people who have a reason to be worried about being infected, or people who are already sick in the hospital. You’ll get more positives from all those people than you would in the general community, so it can be dicey, especially over longer periods of time, to assume that these inflated positivity rates indicate the level of an infection’s spread. In addition, many people who want a test often cannot get one due to long lines, lack of access to free testing, and limitations on who can receive a test in many parts of the U.S.
“Virtually nowhere is doing this random testing of people on the street,” says Hanage. “And as a result of that, the test positivity statistic is almost meaningless in isolation from other things,” including the raw number of people who test positive for the virus.
Indeed, most researchers avoid relying on any single number such as the positivity rate to understand the status of a community’s outbreak, preferring to examine it alongside other statistics, such as the number of and trend direction for positive coronavirus cases in a community — is the number rising or falling? It’s also crucial nowadays in the U.S. to look at these trends in the context of whether local hospitals have available beds, the extent of testing, and the average age at which people get infected, says Boston University epidemiologist Matthew Fox.
“You sort have to make an educated guess,” Fox says. “And I think that’s why there’s so much frustration, because what we want is a scientific approach that tells us that if you hit this number, then it triggers action and we know that that is going to save lives. And we’re just not there. This [virus] is something we’re newly grappling with.”
For instance, it would be misleading to base policy on South Dakota’s 448 new infections reported on December 1 without also looking at its eye-popping positivity rate of 42.5 percent. Together these numbers start to paint a picture of a runaway outbreak and insufficient testing. By contrast, New York state on the same day reported over 16 times more new infections (7,413). In the context of the state’s 3.7 percent positivity rate that day, it could suggest a more controlled outbreak and enough testing to inform efforts to control or respond to transmission. But it is not ideal to base policies on these two figures in the absence of community-wide random testing and other data such as local hospital capacities and available beds, equipment, and staffing.
Youyang Gu, an independent data scientist, has used the positivity rate to estimate the actual or true prevalence of coronavirus infections, pegging the national figure at 16 percent, as of November 18. Without commenting directly on Gu’s work, Fox was cautious about the approach. “To get prevalence, you don’t want people coming to you [for testing] because they have symptoms, or because they have a reason to test. You want to just do a random sample of people,” Fox says.
Meanwhile, the positivity rate statistic is so inconsistently calculated and reported across U.S. states that the COVID Tracking Project, one of the nation’s trusted aggregators and reporters of coronavirus data and trends, doesn’t publish it, says Jessica Malaty Rivera, the science communication lead with the project. An October blog post co-authored by Malaty Rivera called positivity rate figures in the U.S. “a mess” and stated that she and her team “emphatically recommend against over-reliance” on it to justify changes in policy.
And it’s problematic to compare coronavirus positivity rates across communities because calculation methods vary, Malaty Rivera says. Some states take the standard approach, dividing the total number of tests taken by the number of tests that came up positive for the virus. But other states divide the total number of tests taken by the number of people who test positive. That approach gives you lower positivity rates because some people test more than once within a few days, say when they have symptoms or have recently been exposed to someone with the virus. You’re only counting them once in this second approach, but you would count them each time they tested in the standard approach, yielding a higher percentage of positivity.
More recently, COVID Tracking Project data collectors have noticed that states are including the results of less accurate, less expensive so-called antigen tests, which look for pieces of the virus, not the whole virus, instead of the results of widely used PCR tests for the entire virus, Malaty Rivera says.
“For that reason, I feel especially pessimistic about the future of this calculation,” Malaty Rivera says. “Because if we do see testing increase dramatically, it will be because of an influx in antigen testing. It really should just be PCR testing to determine this. And when we combine units, it’s going back to basic fractions, right? You don’t combine your apples and oranges when you’re doing a math equation.”
None of this means we should entirely discard the positivity rate as a statistic. We just need to evaluate it in the context of who is testing and how much testing is conducted in a community, says Fox. If the number of tests performed over a span of two or three weeks remains more or less constant, he says, and the positivity rate increases, it’s reasonable to interpret that more as increased transmission in that community, and not just as an increased shortage of testing.
And to be fair to Mayor de Blasio, that is what the city’s recent positivity data has looked like. Positive test numbers and COVID-19 hospitalizations in the city also have been rising.
Fox says he doesn’t envy decision-makers during the pandemic, given the economic and epidemiologic complexity of the problem and the extremely limited experience all of us have with this coronavirus, beyond the past several months. “We are learning and adapting and learning and adapting,” he says. “And you learn from successes, but you also learn from failures. And there is no easy, right answer in front of us.”