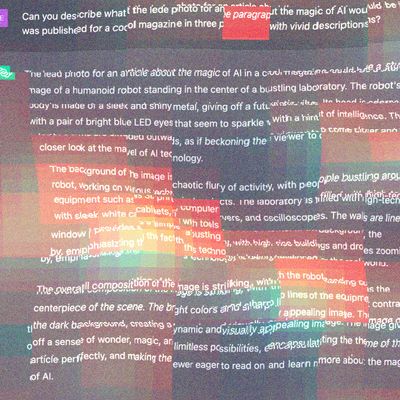
In 2022, artificial-intelligence firms produced an overwhelming spectacle, a rolling carnival of new demonstrations. Curious people outside the tech industry could line up to interact with a variety of alluring and mysterious machine interfaces, and what they saw was dazzling.
The first major attraction was the image generators, which converted written commands into images, including illustrations mimicking specific styles, photorealistic renderings of described scenarios, as well as objects, characters, textures, or moods. Similar generators for video, music, and 3-D models are in development, and demos trickled out.
Soon, millions of people encountered ChatGPT, a conversational bot built on top of a large language model. It was by far the most convincing chatbot ever released to the public. It felt, in some contexts, and especially upon first contact, as though it could actually participate in something like conversation. What many users suggested felt truly magical, however, were the hints at the underlying model’s broader capabilities. You could ask it to explain things to you, and it would try — with confident and frequently persuasive results. You could ask it to write things for you — silly things, serious things, things that you might pass off as work product or school assignments — and it would.
As new users prompted these machines to show us what they could do, they repeatedly prompted us to do a little dirty extrapolation of our own: If AI can do this already, what will it be able to do next year? Meanwhile, other demonstrations cobbled together AI’s most sensational new competencies into more explicitly spiritual answers:
If these early AI encounters didn’t feel like magic, they often felt, at least, like very good magic tricks — and like magic tricks, they were disorienting. It wasn’t just direct encounters with these demonstrations that were confounding, though. Explanations about how deep-learning and large-language models actually work often emphasized incomprehensibility or, to use the terms of art, a model’s explainability or interpretability, or lack thereof. The companies making these tools could describe how they were designed, how they were trained, and on what data. But they couldn’t reveal exactly how an image generator got from the words purple dog to a specific image of a large mauve Labrador, not because they didn’t want to but because it wasn’t possible — their models were black boxes by design. They were creating machines that they didn’t fully understand, and we were playing with them. These models were inventing their own languages. Maybe they were haunted.
Meanwhile, some of the people most responsible for charting the path of AI development — industry leaders like Sam Altman and Elon Musk — continued their breezily provocative debates about if or when machines would become intelligent enough to pose an existential threat to the human species. Would they have mercy? Or are we simply doomed? (During a conference in 2015, before taking charge at OpenAI, Altman quipped, “AI will probably most likely lead to the end of the world, but in the meantime, there’ll be great companies.”) Even from the top, the view of AI was black boxes all the way down.
This was no ordinary next big thing for Silicon Valley. The prevailing narrative of the future of tech — the result of a series of steady breakthroughs in the deeply empirical fields of data science and statistical analysis — seemed to be converging around almost magical discourses. Suddenly it was about forces and phenomena beyond the reckoning of the human imagination. It was about the speedy progress of a technology that, at the deepest level, threatened to alter the terms of our existence in the universe. In the course of a year, the tech industry’s dreary post-social, post-crypto interregnum was rapidly supplanted — largely as the result of public-facing efforts by OpenAI, which is reportedly in talks with Microsoft about a potential $10 billion investment — by a story about inevitable technologies that are so transformative, so incomprehensible, and so unpredictable as to preemptively hurl mankind back into a state of premodern mysticism and awe.
Some of this is creditable to a genuine sense of philosophical upheaval as technologies attempt to address unsettled — and perhaps unresolvable — concepts like intelligence and consciousness. But situating AI as perpetually beyond comprehension is also good for business. In 2020, researchers Alexander Campolo and Kate Crawford termed this dynamic enchanted determinism, which they define as follows:
“A discourse that presents deep learning techniques as magical, outside the scope of present scientific knowledge, yet also deterministic, in that deep learning systems can nonetheless detect patterns that give unprecedented access to people’s identities, emotions, and social character.”
Many breakthroughs in science and technology, however stunning they might first appear, reveal something about how the world works. If they collide with previous (and particularly unempirical) notions about why things are the way they are, they might help produce what the German sociologist Max Weber called a sense of disenchantment — crudely, the result of a process of secularization and rationalization, underpinned by a belief that most, if not all, phenomena are, in theory, explainable.
In and around the AI industry, Campolo and Crawford identified a strange twist on the tendency:
Paradoxically, when the disenchanted predictions and classifications of deep learning work as hoped, we see a profusion of optimistic discourse that characterizes these systems as magical, appealing to mysterious forces and superhuman power.
Crawford elaborated in an interview. “When you have this enchanted determinism, you say, we can’t possibly understand this. And we can’t possibly regulate it when it’s clearly so unknown and such a black box,” she says. “And that’s a trap.” AI models are built by people and trained on information extracted from people. To a far greater extent than they can be said to be incomprehensible or autonomous, they are the product of a series of decisions that informs what they do and why they do it.
Crawford, who recently published a book called Atlas of AI, doesn’t entirely attribute the enchantment of AI to a concerted marketing campaign or credulous journalism, though both are certainly factors. OpenAI, which has been accused by its peers of releasing tools to the public with reckless speed, is particularly good at designing interfaces for its models that feel like magic. “It’s a conscious design imperative to produce these moments of shock and awe,” Crawford says. “We’re going to keep having those moments of enchantment.”
Technological reenchantment is fragile and never lasts long. Thinking and conscious humans are extremely good at taking new technologies for granted, for better or for worse. It won’t take much time for laypeople and experts alike to develop intuitive-enough models of what commercial AI is or does that aren’t clouded by suggestions of magic, rooted in experience rather than speculation: The programming assistant has made my job easier; the programming assistant has made my job more annoying; the programming assistant is a threat to my job — all of the above, eventually.
In the field of AI, encounters with unbelievable chatbots, debates about black-box models, and fears of runaway super-intelligence each have decades of instructive history. In industries where less whimsical modern deep-learning products are already widely deployed — content recommendation, search ranking, mass surveillance — wonder fades fast. The chatbot whose creators can’t explain why it made a particularly funny joke and the social app whose parent company can’t fully explain why it recommended a specific ad are nearly identical stories separated mostly by time; one is the subject of attempts at regulation, and the other is not. (A useful antecedent from another occasionally enchanted industry: Medicines without obvious mechanisms of action are still vetted by the FDA.)
Personified technologies like ChatGPT might even pioneer new sensations of disenchantment — the funniest chatbot in the world will lose its mystique pretty quickly when your employer decides it should make you 45 percent more productive or when it shows up in Microsoft Word dressed as a paper clip.
By the time a new AI tool shows up at your job or decides the size of your mortgage, it’ll be entrenched and harder to challenge. Last year’s AI spectacle, in its overwhelming novelty, has given a few industry players a chance at seizing control. The One Weird Trick for seeing AI clearly, then, is to imagine your inevitable boredom from the start, then trying to figure out what’s left, what it can do for you, and what it wants.
Crawford isn’t dismissive about the potential scale of AI’s various impacts. “We are very much at the beginning of a huge upward curve of a lot of what’s going to happen,” she says. The most significant consequence of a mystical, inevitable account of AI — fostered by clever demos and doomsaying CEOs alike — is that it negates the sort of valid and rigorous criticism that might make it better for the people on whom it will be deployed.
This will be another big year for AI, in other words, and one rich with dazzling new demos that could make ChatGPT seem quaint. Crawford’s book draws from AI’s past and present to sketch a vision of an industry that is material, human, and extractive. Models trained on flawed, biased, and often secret sets of data will be used to attempt to perform an assuredly ambitious range of tasks, jobs, and vital economic and social processes that affect the lives of regular people. They will depend on access to massive amounts of computing power, meaning expensive computer hardware, meaning rare minerals, and meaning unspeakable amounts of electricity. These models will be trained with the assistance of countless low-paid laborers around the world who will correct bogus statistical assumptions until the models produce better, or at least more desirable, outputs. They will then be passed on for use in various other workplaces where their outputs and performances will be corrected and monitored by better-paid workers trying to figure out if the AI models are helping them or automating them out of a job, while their bosses try to figure out something similar about their companies. They will shade our constant submissions to the vast digital commons, intentional or consensual or mandatory, with the knowledge that every selfie or fragment of text is destined to become a piece of general-purpose training data for the attempted automation of everything. They will be used on people in extremely creative ways, with and without their consent.
If prompted, and based on my proprietary model of How Things Seem to Work These Days, trained on, I guess, various things I’ve noticed (don’t ask, not even I can explain), I would guess that we will watch these enchanted and revolutionary tools submit to worldly and conventional priorities of the companies and governments that are funding and deploying them to sometimes great (but often obvious) effect. Efforts to commercialize the new wave of AI will reveal a familiar story about, essentially, a concerted, credible, and well-funded effort to expand the boundaries of automation. That’s no small thing! But it’s exceedingly legible, interpretable, and explainable. AI’s magic will fade. Why not get ahead of it?