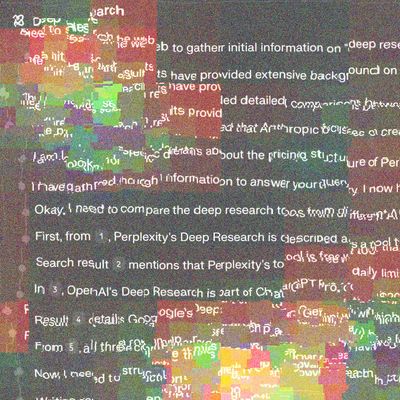
While the immediate post-ChatGPT era was all about chasing OpenAI, the current situation for AI firms is more conventionally competitive. Everyone’s buying the same GPUs to train similar models to attack the same benchmarks; in terms of new features, which closely follow new models, the parallels are not subtle.
Take the sudden arrival of research tools. In December, Google released a tool called Deep Research, which it describes as an “agentic” tool that can “explore complex topics on your behalf and provide you with findings in a comprehensive, easy-to-read report.” In early February, OpenAI announced deep research, which is, in the company’s words, an “agent that can do work for you independently — you give it a prompt, and ChatGPT will find, analyze, and synthesize hundreds of online sources to create a comprehensive report at the level of a research analyst.” A couple of weeks later, Perplexity announced its own feature called Deep Research. A few days after that, Elon Musk came out with a new xAI model and a capability called DeepSearch. These are still (mostly) paid features, but they’ll be commoditized and freely available soon enough.
Their clustered debuts are the result of a few recent shifts and trends in the industry: the recent proliferation of “reasoning” models, which attempt to break down requests into smaller logical tasks; surprising performance gains from increasing test-time compute, which, to oversimplify a bit, means giving AI models more space and processing power to produce answers (in contrast to scaling by simply training bigger and bigger models on more and more data, a technique that was showing signs of slowing down); and the marketing push around AI agents as a bridge to widespread AI job automation, which is the theory undergirding the historically massive levels of investment in the technology. Indeed, these tools try to automate some joblike tasks — note the “research analyst” framing — and do so in a way that’s legible to more of the public than the multistep programming assistance you get from tools like Devin and Cursor. (Experiences with agent-esque programming tools are, I think, an underrated source of enthusiasm for some theories of broad AI acceleration and automation. In situations where outputs can be tested and validated in real time, such tools can work quite well; whether the resulting intuitions are both correct and applicable outside the guardrails of a software-development environment is a trillion-dollar question.)
Like AI programming tools, these deep-research tools show their work. You watch them come up with a plan, and you watch them attempt to execute it. In a broader sense, by doing your Googling and synthesizing and summarizing for you, they purport to show you a different future for consumer AI, less about personified chatting than deployment and supervision.
What will these tools do for you now? Let’s say you ask Google’s Deep Research to perform a task close to home: “Can you compare various ‘research’ tools from AI companies including Google, OpenAI, Perplexity, and xAI?” At first, in my case, it refused, informing me that it couldn’t produce a side-by-side comparison but could instead produce a “helpful report” based on “in-depth research.” Asked to proceed, it produced a short research plan, which I approved. The interface showed its work, generating a list of 19 websites that it was “researching,” producing something akin to a post on an SEO blog: a vague, partial, but reasonably informative skim of the above-mentioned features. It was by no stretch “comprehensive,” which, in terms of avoiding specific errors, probably worked in its favor; still, it ended with a glaring mistake, confusing xAI the company with the field of research known as XAI, or explainable AI, and producing an irrelevant bulleted section about that instead.
Google’s Deep Research is many familiar things at once: an impressive demo that doesn’t stand up to close scrutiny; a preview of a better version of itself that could one day exist but that won’t necessarily get where it’s going. “Not there yet” might be an accurate description, but it could likewise turn out to be a bit too polite. Other tools are already more ambitious, and in some ways better. OpenAI’s Deep Research produces more accurate and comprehensive work, for example, but also makes mistakes that a “research analyst” couldn’t get away with, and fails for reasons that aren’t really analogous to the reasons good-faith human researchers fail:
Whether or not these tools are generally good is sort of beside the point, and a bit of a trap when talking about AI, as are the comparisons they invite to human roles — what matters is whether they end up being economically valuable, and for whom, however they’re deployed or understood. In any case, this is the direction everyone is pushing, with a multi-hundred-billion-dollar tailwind. If things don’t work out, in other words, it won’t be for lack of trying; failure, or merely coming up short of the singularity, will change the world, too.
These tools offer a preview of at least some of that change. Google’s Deep Research report on deep-research tools turned up a lot of basic information as well as a few errors, but unlike the earlier generation of chatbots it also provided citations. The first source it cited was an official OpenAI blog. The second was a blog on Medium titled “In-Depth Comparative Analysis of Deep Research Tools by OpenAI and Perplexity,” published by an AI artist who has generated thousands of images. The post, which is cited multiple times, was almost certainly composed with AI.
A few citations down, we find another Medium blog, this one titled “Comparing Leading AI Deep Research Tools: ChatGPT, Google, Perplexity, Kompas AI, and Elicit.” Not only is this post content marketing for Kompas, “a deep-research and report-generation platform,” but it, too, was almost certainly compiled by AI. While the article has plenty of tells, the associated Medium account publishes a high volume of other articles on a wide range of topics, from politics to music, many including the following disclaimer: “This research and report were fully produced by Kompas AI. Using AI, you can create high-quality reports in just a few minutes.” Deep Research cites two separate posts from this blog, which appears to have little readership. (Under the second article, there is a single comment: “Is this report written by AI?”)
This isn’t just a Google issue: Of the first three sources cited in Perplexity’s report, one sure seems like it was written by AI while another was published on the suspiciously prolific blog of a company called AutoBlogger, which makes AI tools for writing blog posts. It lists 40-plus other sources, including official company sources, reputable newsletters, and news sites; mixed in, however, is plenty of AI-written slop, cited and used in the end product — a report about AI aggregated from reports about AI written by … AI.
Last year, a widely covered paper introduced a theory of model collapse, arguing that models trained on their own outputs would eventually, well, collapse into incoherence, recursively compressing their own outputs into fuzz. It was intuitive to a lay-reader and posited an appealing, just destiny for AI firms whose tools were both trained on the web and seemed to be filling it with slop. It also turned out to be relatively easy to mitigate: As long as models are trained on a mixture of real and synthetic data, they can avoid collapse. Likewise, the fact that these new AI research-report tools already appear to be citing AI-generated “research reports” teases recursive doom in the era of reasoning models and agents, in which these multibillion-dollar machines could end up with nothing left to aggregate but their own recycled outputs.
It’s safe to say that companies like Google, OpenAI, xAI, and Perplexity are aware of this and working to obtain access to data beyond the decaying open web, either through licensing deals (disclosure!) or less transparent means. It’s also worth considering that recycled outputs might function as a sort of commodity-grade synthetic data, mingling with other sources in a way that’s useful, or at least not destructive, to AI firms. In the above example reports, the AI-generated sources were derivative and contained some errors, but they were, as part of a mixture of sources, plausibly good enough for the task at hand, which could be to produce a document good enough to start researching, or, more cynically, to file into a workflow where it was likely to be ignored anyway. They had value to Google: They weren’t hidden behind paywalls, nor were they likely to generate lawsuits. The best way I can describe these reports is as a form of laundered content — full of technically untraceable material, the origins of which are nonetheless obvious to anyone who cares.
For the rest of the web — and for anyone who fills it with content for fun, friendship, or profit — the situation is less obviously salvageable. One way to think about the big technological shift in AI in the past few months is that AI companies are figuring out how to get more value out of the data and systems they’ve already accumulated and built. These deep-research tools demonstrate what this second round of extraction looks like in product form. First, AI companies scraped the entire web to build generative machines. Then, people started using those machines, filling the web with approximations of the scraped material. Now, those machines are being sent back to scrape the web again, occasionally encountering their own waste product in the wild, all on the way to a glorious if ill-defined post-web, post-human future.
The success of Google’s search engine expanded access to the web and helped grow its loose economy; over time, it also remade the web in the company’s image and ordered it according to the company’s advertising logic. (One striking thing about watching Google’s Deep Research tool work is seeing anew how utterly poisoned the web is by pre-generative-AI SEO spam, and how completely obvious the problem is when you remove it from Google’s familiarly disorienting search interface.) With Search there was a bargain, however corrosive it might have ultimately been: You provide us with things to “Search” for, and we’ll help you make money with traffic and ads. These deep-research features, and generative AI tools in general, promise no such exchange. They hardly lead anyone anywhere — they simply take what they want and leave. (Similarly lopsided arrangements are implied in many pitches for other sorts of AI agents, which promise to carry out tasks on behalf of their users, and in some cases as their users, with little concern for what that means for the environments in which they’ll work and the other parties with which they’ll interact.)
Seen through the lens of AI training and automated research, the web isn’t a human commons to be preserved or even joined with the intent of exploitation. It’s more external than that: a vulnerable resource to be mined and mined again until it’s not needed anymore or there’s nothing of value left, whichever comes first. The web as we know it — the strange, old, sprawling collection of deliberate human output and collected human behaviors — is in this view a mass of raw material that AI firms think they can use to get where they want to go. From their perspective, it doesn’t matter much what they leave behind.
More screen time
- Is Google Eating Reddit?
- Big Tech’s Other Big Battle Is for the Box
- The DOGE Rampage Is Also a Bailout for Elon Musk